- 1Programa Institucional de Doctorado en Ciencias Agropecuarias y Forestales, Universidad Juárez del Estado de Durango, Durango, Mexico
- 2School of Forestry, Northern Arizona University, Flagstaff, AZ, United States
- 3Instituto Politécnico Nacional, Durango, Mexico
- 4Instituto de Silvicultura e Industria de la Madera, Universidad Juárez del Estado de Durango, Durango, Mexico
- 5SMartForest Group, Department of Biology of Organisms and Systems, Mieres Polytechnic School, University of Oviedo, Mieres, Spain
- 6Facultad de Medicina Veterinaria y Zootecnia, Universidad Juárez del Estado de Durango, Durango, Mexico
Insect damage to cones and seeds has a strong impact on the regeneration of conifer forest ecosystems, with broader implications for ecological and economic services. Lack of control of insect populations can lead to important economic and environmental losses. Pinus strobiformis is the most widespread of the white pines in Mexico and is widely distributed throughout the mountains of northern Mexico. Relatively few studies have examined insect damage to the cones and seeds of these pines, especially in Mexico. In this study, we therefore analyzed insect damage to cones and seeds of P. strobiformis in Mexico by using X-ray and stereomicroscopic analysis. The specific objectives of the study were (a) to characterize insect damage by measuring external and internal cone traits, (b) to assess the health of seeds and cones of P. strobiformis in the Sierra Madre Occidental, Mexico, and (c) to estimate the relative importance of the effects of different environmental variables on cone and seed damage caused by insects. We found that 80% of P. strobiformis seeds and 100% of the tree populations studied had damage caused by insects. Most seeds were affected by Leptoglossus occidentalis, Tetyra bipunctata, Megastigmus albifrons, and the Lepidoptera complex (which includes Apolychrosis synchysis, Cydia latisigna, Eucosma bobana, and Dioryctria abietivorella). The cones of all tree populations were affected by some type of insect damage, with Lepidoptera causing most of the damage (72%), followed by Conophthorus ponderosae (15%), the hemipteran L. occidentalis (7%), and the wasp M. albifrons (6%). The proportion of incomplete seeds in P. strobiformis at the tree level, cone damage by M. albifrons and seed damage in L. occidentalis were associated with various climate and soil variables and with crown dieback. Thus, cone and seed insect damage can be severe and potentially impact seed production in P. strobiformis and the reforestation potential of the species. The study findings will enable managers to better identify insects that cause damage to cone and seeds. In addition, identification of factors associated with damage may be useful for predicting the levels of insect predation on seeds and cones.
Introduction
Insect damage to tree cones and seeds is an important factor (Bramlett et al., 1977; Hedlin et al., 1980) affecting the regeneration of conifer forest ecosystems and also has broader implications for ecological and economic services (Wickman, 1992). Large economic and environmental losses can be generated if insect populations are not adequately controlled (Ruth et al., 1982; Dormont et al., 1996).
Multiple insect orders affect both the cones and seeds of several Mexican pines (Hedlin et al., 1980; Cibrián-Tovar et al., 1986); previous studies identified 49 insect species, belonging to six orders and 11 families, which attack cones and seeds of conifers in Mexico (Del Río-Mora, 1980; Hedlin et al., 1980; Cibrián-Tovar et al., 1986, 1995). Cone infestation and damage can reach high levels in natural forests. According to Blake et al. (1989), mortality rates reached between 38 and 81% in Arizonan Pinus ponderosa Doug. cones in 1984, as a result of damage caused by Dioryctria auranticella Grote (Lepidoptera: Pyralidae); damage by Conophthorus ponderosae Hopkins (Coleoptera: Scolytidae) also provoked cone mortality of between 3.9 and 36.4 %, while Megastigmus albifrons Walker (Hymenoptera: Torymidae) caused death of between 46 and 70% of seeds.
The insects known to damage the cones and seeds of Mexican conifers belong to seven orders, Coleoptera: represented by cone beetles in the genus Conophthorus spp. (Curculionidae: Scolytinae) are widely distributed and highly destructive to Mexican pine seed, and the cone weevil, Conotrachelus neomexicanus Fall. (Curculionidae: Molitinae) (Cibrián-Tovar et al., 1986). The order Hemiptera: include other relevant and harmful pests such as the western conifer seed bug, Leptoglossus occidentalis Heidemann (Hemiptera: Coreidae), and the shieldbacked pine seed bug, Tetyra bipunctata, Herrich-Schäffer (Hemiptera: Pentatomidae). Other pests of importance include the Hymenoptera: seed wasps, M. albifrons Walker (Torymidae). The Lepidoptera: such as cone borers, Dioryctria, Eucosma, and Cydia, is a genera with relevant species to cones of most of the Mexican pines. In the order Diptera: the southern cone gall midge, Cecidomyia bisetosa Gagné and the cone resin midge, Contarinia spp. and Asynapta spp. Felt (both, Cecidomyiidae), are important species that cause damage to cones. Homoptera: Chionaspis pinifoliae Fitch may become a pest of relative relevance for Mexican pines, covering some part of developed cones. Thysanoptera: has some species, such as Haplothrips spp. (Phlaeothripidae) that causes damage to conelets (Hedlin et al., 1980; Ruth et al., 1982; Cibrián-Tovar et al., 1986; Álvarez-Zagoya and Márquez-Linares, 1994a,b; Fairweather et al., 2006; Salinas-Moreno et al., 2010; Bustamante-García et al., 2012; DePinte et al., 2020).
T. bipunctata causes partial damage to second year seeds. But L. occidentalis leaves empty or almost totally empty seeds in second year cones (Hedlin et al., 1980; Cibrián-Tovar et al., 1986). DeBarr (1970) and Bramlett et al. (1977) reported that the type and size of seed damage depend on the size of the oral apparatus of the seedbugs, since there is a greater or lesser penetration through the layers of the scales. In L. occidentalis, if feeding occurred during the second year of cone development, a small feeding scar is evident on the mature seed covers (DePinte et al., 2020).
X-ray analysis is a non-destructive, rapid method that is commonly used to analyze conifer seed health (DeBarr, 1970; Bramlett et al., 1977; Chavagnat, 1985; Lippitt et al., 1994; Machado and Cícero, 2003; Himanen et al., 2015). It has been used to study several pine species, including Pinus banksiana Lamb., Pinus elliottii Engelm., Pinus palustris Mill., Pinus ponderosa Laws., Pinus echinata Mill., Pinus taeda L., Pinus virginiana Mill., Pinus strobus L., Lithraea molleoides (Vell.) Engl. and Picea abies L. Cone damage in pines is also assessed by other methods, like measurement of dissected cones scale by scale (Blake et al., 1989), cone measurements depending on sampling date, age and damage category (Bracalini et al., 2013), and determining the percentage of each type of damage in cones of Pinus ponderosa Douglas, Pinus cembra L., Pinus albicaulis Engelm, Pinus pinea and P. strobiformis Engelm. (Schmid et al., 1984; Dormont et al., 1996; Kegley et al., 2001; Bracalini et al., 2013; Linhart et al., 2014; DePinte et al., 2020).
Insect populations are strongly affected by deforestation and climate variation (Dunn and Crutchfield, 2006). High reproduction rates may not occur if occasional high temperatures prevent survival until maturity of insects in temperate and tropical regions (Kingsolver et al., 2011). Klapwijk et al. (2013) concluded that Lepidoptera species are positively affected by some changes in weather conditions (increased temperature and precipitation). In other species of insects, such as some members of the order Heteroptera, the increase in average summer temperature and decrease in the winter temperature can improve the potential conditions for hibernation and enable the species to extend to wider ranges, where they were not previously found (Musolin, 2007). In a study of the distribution of invasive insect populations in New Zealand, a high proportion of sites where some insects are present were found to be correctly classified by temperatures in late spring and early summer (Peacock et al., 2006). Moreover, several studies reported that certain soil conditions may be correlated with distribution, seasonal activities. and abundance of insects (e.g., McColloch and Hayes, 1922; Choudhuri and Banerjee, 1975) and insect attacks (Altieri and Nicholls, 2003).
Regression models are commonly used to predict probabilities of insect attacks and damage as a function of environmental factors and specific features of stands and individual trees (e.g., Negrón et al., 2009) and to harness scientific knowledge for effective ecosystem management (Seidl et al., 2011). Several comprehensive models of forest susceptibility to insect attack and damage were reported, such as by Luther et al. (1997) for Acleris variana Fern. and Wulder et al. (2006) for Dendroctonus ponderosae Hopk. particularly, however, machine learning algorithms demonstrated high capacity to predict physical condition of trees associated with insect damage, such as to forecast tree death or survival following the attack of Thecodiplosis japonensis Uch. et Inou where artificial neural networks (Park and Chung, 2006) were used to predict potential sanitary fellings of bark beetle-attacked Norway spruce, based on 21 climate, soil, and forest variables through multivariate regression trees (Ogris and Jurc, 2010) and also to estimate defoliation of Scots pine stands in western Poland, by k-nearest neighbors, random forest, and support vector machines as well as Sentinel-2 vegetation indices (Hawryło et al., 2018). White pines (genus Pinus, subgenus Strobus) are common tree species in temperate forest ecosystems. These species provide wood, resin, and pulp as well as valuable ecological services, such as provision of wildlife habitat (Farjon and Styles, 1997; Richardson, 2000; Villalobos-Arámbula et al., 2014). White pine seeds are large and nutritious and provide a valuable food source for rodents, parrots and other birds (Samano and Tomback, 2003; Looney and Waring, 2013). Conserving these pine species and their genetic diversity is therefore of great ecological importance (Bower et al., 2011). P. strobiformis is the most widespread of the white pines in Mexico and is widely distributed throughout the mountains of northern Mexico, from northern Chihuahua to the state of Jalisco in the south (Shirk et al., 2018; Leal-Sáenz et al., 2020).
P. strobiformis is currently facing multiple threats; e.g., range distribution models indicate that distribution of this species will contract in Mexico by up to 60% by 2080, due to increasingly unfavorable climate conditions (Shirk et al., 2018). The species is also highly susceptible to white pine blister rust, an invasive tree disease caused by the fungus Cronartium ribicola J. C. Fisch. (Geils et al., 2010), which occurs in the southwestern United States (not yet found in Mexico). Moreover, insect damage to P. strobiformis could exacerbate any future decline in the species (Looney and Waring, 2013; DePinte et al., 2020).
Relatively few studies have examined insect damage to cones and seeds of P. strobiformis, especially in Mexico (see Cibrián-Tovar et al., 1986, for general information and DePinte et al., 2020, for a similar study in the southwestern United States). In this study, we therefore analyzed insect damage to the cones and seed of P. strobiformis in Mexico. The specific study objectives were as follows: (a) to characterize insect damage based on external and internal cone traits; (b) to assess the health of seeds and cones of P. strobiformis in the Sierra Madre Occidental, Mexico; and (c) to estimate the relative importance of the effects of different environmental factors on cone and seed damage caused by insects. We expect the study findings to help us understand more about the environmental factors contributing to the probability of detecting particular insect species and also to enable us to estimate the damage in the sampled populations.
Materials and Methods
Species and Study Sites
In Mexico, P. strobiformis is distributed in the state of Sonora, western Chihuahua and Durango, and northern Jalisco and Zacatecas (Figure 1; Looney and Waring, 2013; Villagómez-Loza et al., 2014). P. strobiformis mainly occurs at elevations of between 2,500 and 3,000 m, with a mean daily downwelling shortwave solar radiation of 15 kW m−2 (Aguirre-Gutiérrez et al., 2015).
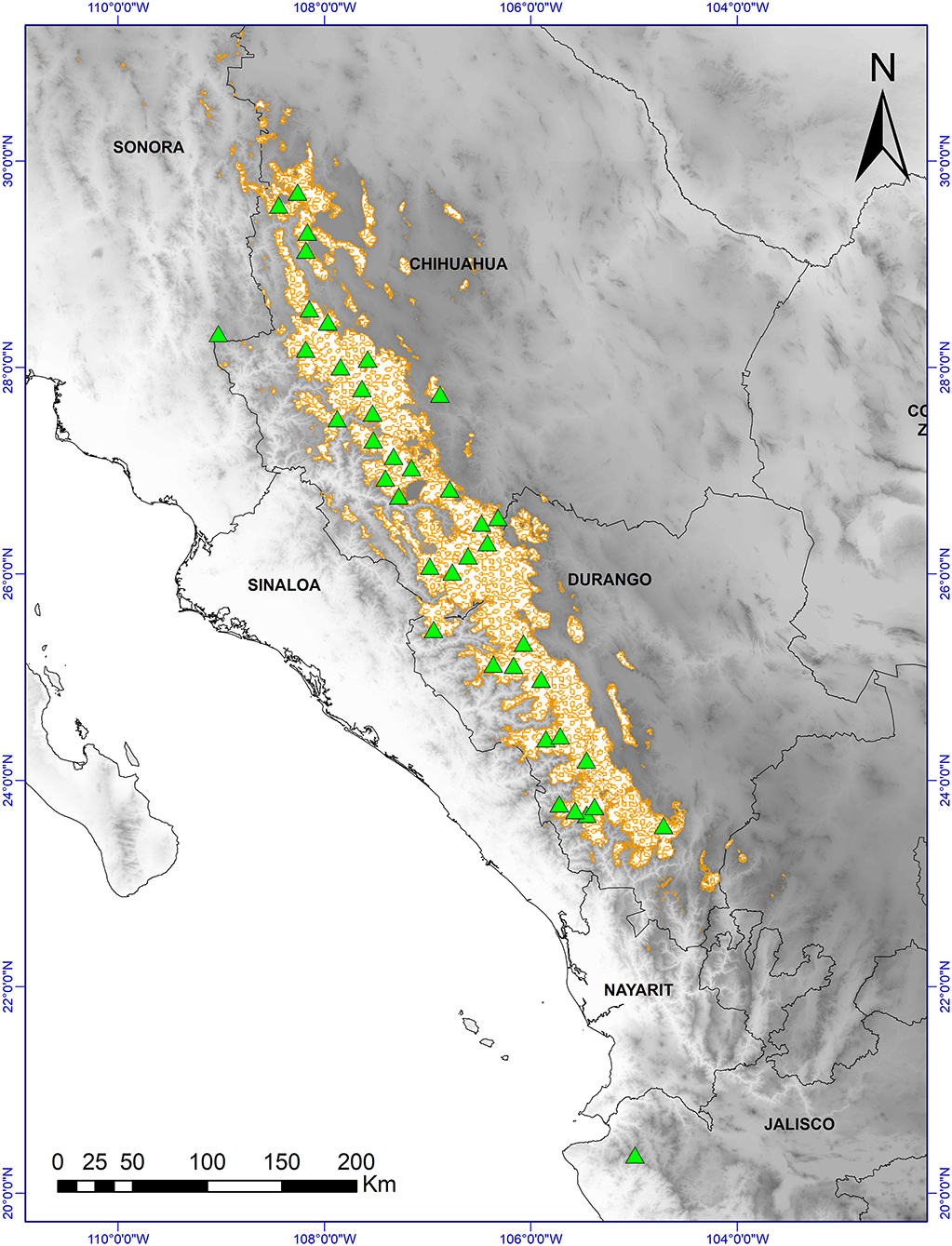
Figure 1. Distribution of Pinus strobiformis (brown outlined areas) and sample collection sites: populations (x40) (green triangles) in which 192 trees were sampled; distribution map based on Shirk et al. (2018). Base digital elevation map from Jarvis et al. (2008).
In 2015, we collected cones and seeds from 40 P. strobiformis populations (192 trees) (Figure 1). The minimum distance between sites was 50 km, and individual trees were separated by a minimum distance of 50 m. The stands were located in closed forests, with minimal human disturbance. Three to five trees with mature cones were selected for study in each population, collecting at least 15 cones per tree (total 4,455 cones), which were also used for another study. The cones were randomly chosen from the upper third of the crown of each tree studied and all seeds were taken from these cones (Supplementary Table 1).
Data Collection
Cone and Seed Damage by Insects
X-Ray Scanning of Seeds
In order to observe damage inside seeds, then, 10 seeds were randomly chosen per tree (i.e., total 1,920 seeds) and were X-rayed using Faxitrion X-Ray Corp. Model MX-20 2006 ® equipment. The X-ray was used to produce an image (tagged image file format, TIFF) of these seeds.
All seeds were examined to evaluate whether they were affected by insect damage, and to identify the type of damage, according to the morphological characteristics of the insect and previously published reports (Bramlett et al., 1977; Hedlin et al., 1980; Ruth et al., 1982; Cibrián-Tovar et al., 1986; Álvarez-Zagoya and Márquez-Linares, 1998; Bustamante-García et al., 2012; DePinte et al., 2020). Damage severity was assigned using shape and color coding (Bustamante-García et al., 2012) in the IMAGE J program (Table 1). As the damage caused by the four species of Lepidoptera reported here was very similar, it was reported as a single factor (Table 1; Figure 2). Finally, we created an identification guide to insect damage on X-ray seeds.
Assessment of Cone Damage
Five cones per site (i.e., total 200 cones) were randomly chosen from the collected cones to identify insect damage to the outer and inner parts of the cone. One year after collection, the cones were cut lengthwise through the middle section, with a metal saw (Supplementary Table 1).
A Canon Power Shot A640 camera was used to capture an exterior image of each half of each cone, and each image was enlarged four times. Insect damage was then evaluated according to previous guidelines (Hedlin et al., 1980; Ruth et al., 1982; Cibrián-Tovar et al., 1986; Bustamante-García et al., 2012; DePinte et al., 2020). The damage was examined under a stereomicroscope (Zeiss stemi 2000C with an ocular magnification of 10X and objective magnification of 6X) (Figure 3).
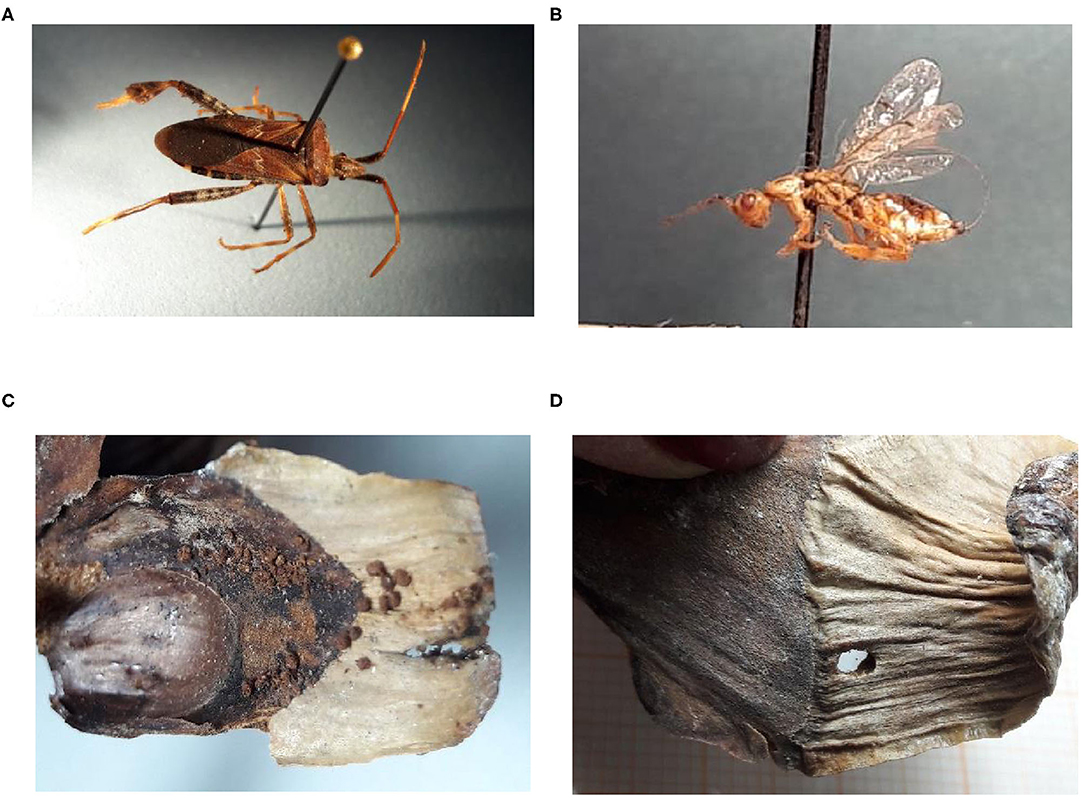
Figure 3. Examples of insects and the damage they cause in cones of Pinus strobiformis: (A) adult Leptoglossus occidentalis (Heidemann) (Photo: Álvarez-Zagoya, 2019), (B) adult Megastigmus albifrons (Walker) (Photo: Álvarez-Zagoya, 2019), (C) damage with frass by Lepidoptera [Apolychrosis synchysis (Pogue), Cydia latisigna (Miller), or Eucosma bobana (Kearfott), Dioryctria abietivorella (Grote)], (D) damage by Conophthorus ponderosae (Hopkins).
Geographic, Topographic, and Dendrometric Data
For each sample tree, the latitude, longitude, elevation (m) and geographical aspect (0–360°) were recorded: for further analysis, the geographical aspect (0–360°), position of the slope (%), disease damage (dieback, shoot death, and leader condition), diameter at breast height (DBH, 1.3 m, recorded in cm), total tree height (m), height to the base of the live crown (m), and first live branch height (m) were determined (Table 2). The geographical aspect was transformed into a cosine index (as 0° and 360° have the same zenithal aspect) (Cushman and Wallin, 2002).
Site Soil Analysis
In each of the 40 sites, a soil sample was obtained at a depth of 15 cm, which has been recommended in forest soil surveys manual (CONAFOR, 2011; Mendoza and Espinoza, 2017). Four soil subsamples (each 250 g) were collected at the base of four P. strobiformis trees studied and were mixed to make a composite sample of 1,000 g for each site.
In total, 27 soil variables were analyzed. Texture (relative proportions of sand, silt, and clay), density (Den) (g/cm3), concentration of calcium carbonate (CaCO3) (%), pH (CaCl2, 0.01 M), concentrations of potassium (K) (ppm), magnesium (Mg) (ppm), sodium (Na) (ppm), copper (Cu) (ppm), iron (Fe) (ppm), manganese (Mn) (ppm), zinc (Zn) (ppm), and calcium (Ca) (ppm) in the soil were determined by the methods described by Castellanos et al. (1999). Phosphorus (P) (ppm) was determined by the method of Olsen et al. (1954), while nitrate (NO3) (kg /ha) was determined by the method of Baker (1967), and the relative organic matter (OM) content (%) was determined by the method of León and Aguilar (1987). Electrical conductivity (CE) (dS/m) was determined by the method described by Vázquez and Bautista (1993). The cation exchange capacity (meq 100 g soil) (CEC) and the relative proportions (%) of hydrogen, Ca, M, K, Na, and other bases (o.b.) in the CEC were estimated on the basis of the Ammonium Acetate Method (pH 8.5) (Knudsen et al., 1982; Lanyon and Heald, 1982). The hydraulic conductivity (HC) (cm/h) was determined by the method of Mualem (1976), and percent saturation (Sat) (%) was estimated by the method of Herbert (1992). The soil analyses are summarized in Supplementary Table 2.
Occurrence of Woody Plants
At each site, we also recorded the following biotic factors: occurrence of the regeneration of Pinus strobiformis, presence of Ribes spp. (alternative host of white pine blister rust) and the presence of woody species in the neighborhood (Cupressus, Pinus lumholtzii, Pinus arizonica, Pinus cooperi, Pinus durangensis, Pinus engelmannii, Pinus leiophylla, Pinus pseudostrobus, Pinus strobiformis, Pinus teocote, Quercus fulva, Quercus sideroxyla, Arctostaphylos pungens, Juniperus deppeana, Arbutus xalapensis, Castilleja angustifolia, Montanoa grandiflora, Filicopsida). The presence of these species was recorded within a 20 m radius of each P. strobiformis sample tree. The occurrence data were transformed into frequency of occurrence prior to analysis (Supplementary Tables 3, 4). Climatic data (20 variables of temperature and precipitation) were downloaded from the PRISM database administered by the University of Idaho, USA (http://forest.moscowfsl.wsu.edu/climate/) (Supplementary Table 5).
Data Analysis
To reach our objective of estimating the relative importance of the effects of different environmental factors (see Supplementary Table 5) on cone and seed damage, caused by the insects studied, we used statistical techniques to select those environmental independent factors that best predicted such damages and computed models for cone and seed damages based on those factors.
Feature Selection and Parametrization of Independent Environmental Factors Influencing Insect Damage
Adequate selection of significant independent variables influencing insect damage in the seeds and cones of 192 P. strobiformis trees was conducted by a Kruskal-Wallis test (KW), partial least squares (PLS) and the univariate curve of the receiving operator (ROC) (Krzanowski, 1987; Gnanadesikan et al., 1995; Maronna et al., 2018). The non-parametric Kruskal-Wallis test (Kruskal and Wallis, 1952) was applied to elevation and latitude data (Supplementary Tables 1–5).
The PLS and the univariate curve of the ROC with the “varImp” function was used along with the machine learning algorithm Random Forest (version 3.3.4; R Development Core Team, 2017). The weighted sums of the weights were determined with a function of the reduction in sum of squares with the PLS components (Kuhn, 2012).
The non-parametric Spearman correlation coefficient (rs) was used to determine collinearity between the significant independent variables of the 192 P. strobiformis trees, as indicated by the Kruskal-Wallis test, PLS or ROC. When the absolute value of the correlation coefficient between two variables was >0.70, only the variables with the lowest p-value were included in the models (as reported by Salas et al., 2017 and Shirk et al., 2018). Finally, all significant variables without collinearity (36 variables) from 78 mostly non-normally distributed independent variables (α = 0.01) were included in subsequent analyses.
Modeling Spatially Dependent Insect Damage Traits by Machine Learning Algorithms
To predict the most spatially dependent P. strobiformis cone and seed traits for selected important, independent variables of the 192 P. strobiformis sample trees, we used machine learning models, since they are fast, efficient and accurate for large-scale analyses (Badnakhe et al., 2018).
The number of variables for these models was determined by the rule of 10 events per variable (McGarigal et al., 2000; Vittinghoff and McCulloch, 2007: i.e., a maximum of the eight most important variables from the 192 trees was included in the models). These regression models, including five-fold cross-validations, were implemented in the “caret” package and function “train”: (i) linear regression (method = “lm”); (ii) Random forest (method = “rf”); (iii) Neural network (method = “nnet”); (iv) Average neural network model (method = “avNNet”); (v) Multilayer perceptron (method = “mlpWeightDecay”); and (vi) Bayesian Regularized Neural Networks (method = “brnn”) (Venables and Ripley, 1999; Williams et al., 2018, http://topepo.github.io/caret/index.html) in R (version 3.3.4) (R Development Core Team, 2017).
(Multiple) Linear regression is one of the simplest machine learning algorithms, often used for comparison purposes and creating parametric regression equations based on two (or more) parameters (Leal-Sáenz et al., 2020). A non-parametric Random Forest regression is based on constructing several decision trees at training time and outputting the average of the classes as the prediction of all individual trees (Breiman, 2001). A neural network is a series of algorithms intended to detect underlying relationships in a data set, mimicking the functioning of the human brain. A neural network comprises layers of interconnected model neurons (perceptrons) corresponding to multiple linear regressions. The model neuron sends the signal generated by multiple linear regression into an often non-linear activation function (see more in Krogh, 2008). We used Random Forests and Neural Networks because they have previously been applied in analysis of forest damages, and they have been found to perform better for events (especially in forestry) than other statistical methods (Hanewinkel, 2005; Guo et al., 2016). These seven algorithms were also successful to predict seed and cone traits of P. strobifomis by environmental variables (Leal-Sáenz et al., 2020).
The goodness of fit of the regression model was evaluated using the (pseudo) coefficient of determination (R2), the root mean square error (RMSE), and the mean squared error (MSE).
Results
Assessment of Seed and Cone Damage
We designed an identification guide that includes different categories of seed damage in P. strobiformis: incomplete, empty and malformed seeds, as well as damage caused by several types of insect (Figure 2). Representative damage by L. occidentalis and the Lepidoptera complex can be observed on the outside and inside of the seed. In M. albifrons and T. bipunctata, the damage is internal only.
Another identification guide for evaluating insect damage in cones of P. strobiformis was created using the data and images from the sites analyzed. Examples of cone damage by insects are described in the Figure 3. Excrement, silk threads, helical tunnels and reddish brown coloration in cones were typical types of frass produced by the Lepidoptera complex. On the other hand, C. ponderosae entered the subcortical cut and dug a tunnel along the axis of the cone, leaving a hole of approximately 2 mm at the entrance; the attack occurred in the basal third of the cone.
We found that 100% of the populations studied and 80% of P. strobiformis seeds had been damaged by insects (Figure 4). Most seeds were affected by the Lepidoptera complex as well as M. albifrons, L. occidentalis, and T. bipunctata. Although the last two species were found in all the populations studied, M. albifrons was only detected in 25% of the populations and the Lepidoptera complex in 73%. Only 20% of seeds in all the populations studied were undamaged or showed some other type of damage, not caused by insects (Figure 4).
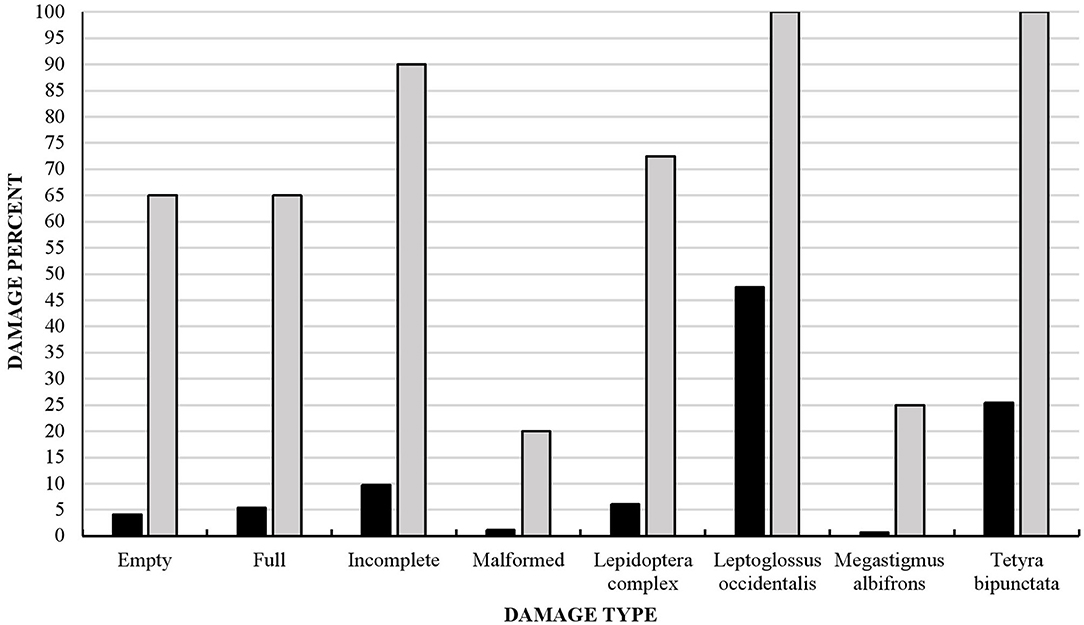
Figure 4. Insect damage in 1,920 seeds from 40 Pinus strobiformis populations sampled and studied at the seed (black) and insect population level (gray).
All tree populations had some type of cone damage caused by members of the Lepidoptera complex, which was the most representative type (72% of damage) (Figure 5), followed by C. ponderosae (15% of damage), the hemipteran L. occidentalis (7% of damage), and the wasp M. albifrons (6% of damage).
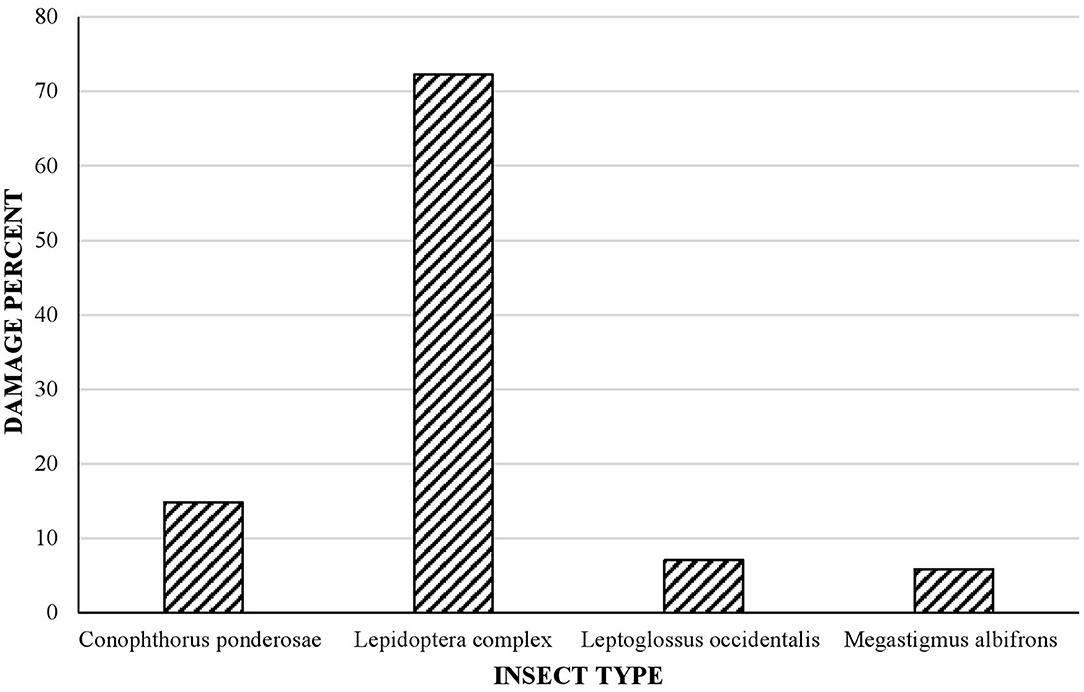
Figure 5. Percentage of cones damaged by different types of insect, determined in five randomly chosen cones per tree population studied (40 populations, total 200 cones). Note that some cones were damaged by more than one insect species.
Modeling Seed and Cone Traits and Insect Damage in Relation to Environmental Variables by Using Regression Machine Learning Algorithms
The independent variables that together produced the best model to estimate incomplete seeds in P. strobiformis at tree level were the degree-days >5°C (DD5, degree-days), growing season precipitation (GSP, mm), winter precipitation (WINP, mm), summer precipitation balance (SMRPB, mm), manganese concentration in the soil (Mn, ppm), crown dieback (a measure of tree health), potassium concentration in the soil (K, ppm), and the proportion of Mg in CEC (MgCEC), yielding an RMSE of 0.115 (proportion of damage) using the Bayesian Regularized Neural Networks (brnn) (Table 3; Supplementary Figure 1). The second-best model for estimating incomplete seed at tree level [RMSE = 0.118 (damage proportion)] was produced by the neural network (nnet) and included the following variables: mean temperature in the warmest month (MTWM, degrees C), SMRPB, dieback, GSP, percent saturation in the soil (%Sat), zinc concentration in the soil (Zn, ppm), Mn, and length of the frost-free period (FFP).
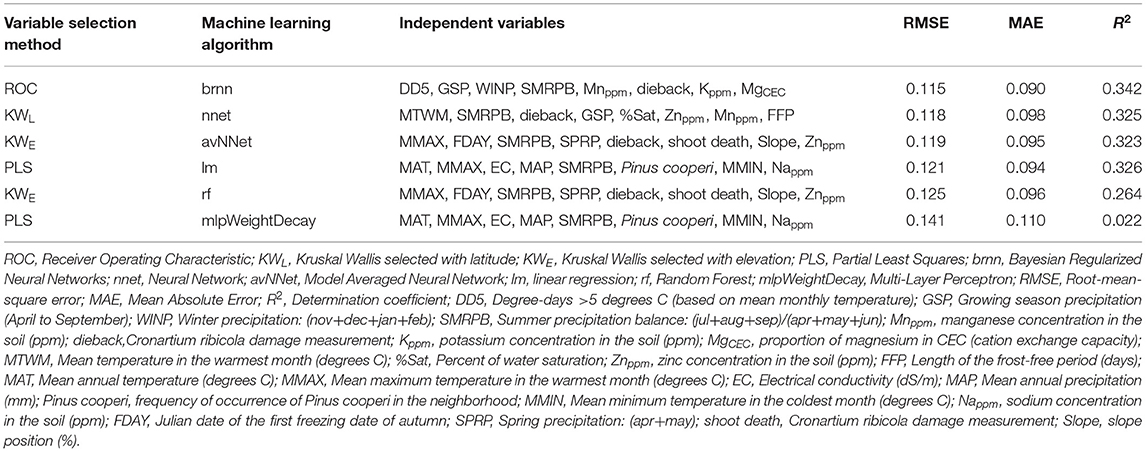
Table 3. Best fit models for estimating the percentage of “incomplete seed” damage, based on the sample of 192 Pinus strobiformis trees.
By contrast, MTWM and the regeneration of P. strobiformis, as well as several soil variables, including OM content (%), Zn, Mg in CEC, Cu concentration (ppm), and Hydraulic conductivity (HC, cm/h), were the variables that together provided the best prediction of the presence of cone damage in P. strobiformis by M. albifrons at population level (RMSE = 0.009) by using the Bayesian Regularized Neural Networks (brnn). The second best model for estimating the presence of cone damage percentage by the same insect (with RMSE = 0.010) was produced using the same variables and Random forest (rf) (Table 4; Supplementary Figure 2).
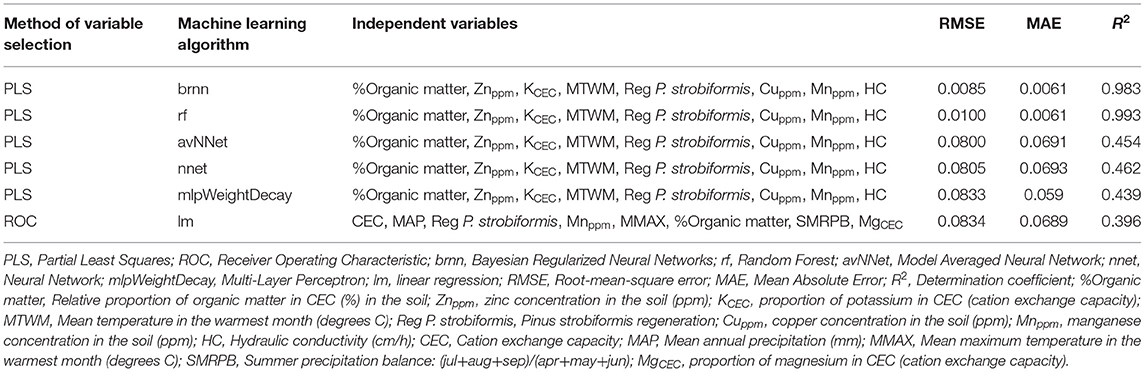
Table 4. Best fit models for estimating the percentage of cones damaged by Megastigmus albifrons, based on the sample of 192 Pinus strobiformis trees.
By contrast, SMRPB, DD5, WINP, Mn in the soil, KCEC, GSP, and the frequency of occurrence of Arbutus xalapensis and Juniperus deppeana in the neighborhood together provided the best prediction of seeds damaged in P. strobiformis by L. occidentalis at the tree level [with an RMSE of 0.199 (damage proportion) with the Model Averaged Neural Network (avNNet)]. The second best model of seed damage by L. occidentalis at tree level [RMSE = 0.201 (damage proportion)] was produced using the same variables and the Bayesian Regularized Neural Networks (brnn) approach (Table 5; Supplementary Figure 3).
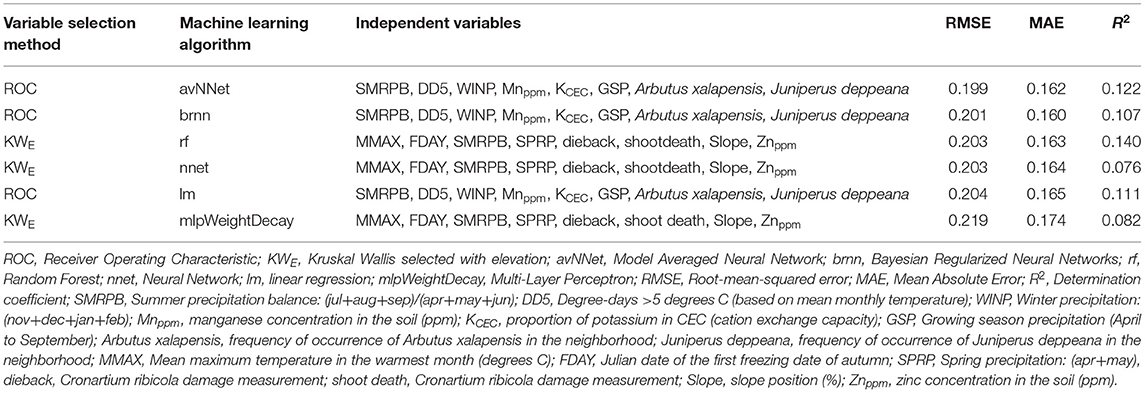
Table 5. Best fit models for estimating percentage of seeds damaged by Leptoglossus occidentalis, based on the sample of 192 Pinus strobiformis trees.
The SMRPB was negatively correlated with the percentage of incomplete seeds (Supplementary Table 6). The percentage of cones damaged by M. albifrons was positively correlated with the OM content (%) of the soil and negatively correlated with the regeneration of P. strobiformis and Mn in the soil (Supplementary Table 7). Seed damage by L. occidentalis was positively correlated with SMRPB, DD5, WINP, Mn, KCEC in CEC and negatively correlated with GSP and the frequency of occurrence of A. xalapensis and J. deppeana in the neighborhood (Supplementary Table 8).
Discussion
Characterization of Insect Damage
We found a wide variety of insect damage in seeds and cones of the Mexican P. strobiformis populations studied, reported here for the first time. Our guide to identification of the seed and cone damage will help managers to characterize damage, identify causal agents and will also help in monitoring seed and cone damage by insects. Identifying the damaging agent is important as insect abundance and distribution are likely to vary across the range of P. strobiformis (DePinte, 2016). Identifying the insects that cause the damage, the locations involved and the proportion of the cone and seed production for a given location is critical for predicting available seed for reforestation and proactive management. While seed and cone insects comprise one of the least understood insect guilds in regard to climate change interactions, the relationships between insects and hosts will probably change (Pureswaren et al., 2018). High levels of insect damage to cone and seed production could greatly reduce the regeneration potential and also the availability of healthy seeds for use in seedling production, research and tree breeding programs (Hedlin et al., 1980; Cibrián-Tovar et al., 1986).
Several of the damage indicators we report here have previously been found in other host species. Bracalini et al. (2013) reported resin excess as an indication of L. occidentalis attack, along with the interaction with several other insects present in cones and seeds of P. pinea in Italy. The Lepidoptera complex insect indicators, including agglomeration of frass, pupae, and a reddish coloration, reported in previous research (Hedlin et al., 1980; Cibrián-Tovar et al., 1986; Whitehouse et al., 2011), were also observed in the present study.
Assessment of Seed and Cone Health
We observed a high percentage of insect damage in P. strobiformis seeds and cones at both seed and population levels. However, Kelly and Sork (2002) reported that cone and seed production are adaptive traits that are spatially and temporally variable, with several years of cone and seed production driven by both predation avoidance and pollen dispersal. Insect predators and their host trees have closely linked developmental stages, leading to fluctuations in insect populations that generally follow oscillations in cone and seed production (Turgeon et al., 1994; Poncet et al., 2009). Our results from a single year observations provide insight into the potential for damage but, indeed, additional research is needed to reveal long-term spatial and temporal patterns of insect abundance, distribution, and population dynamics and the consequent damage to P. strobiformis reproductive potential.
Leptoglossus occidentalis was found to cause the most damage in the present study, followed by Tetyra bipunctata. Previous research in Pseudotsuga mensiezii found that light to moderate feeding by L. occidentalis reduced seed germination by 80% (Bates et al., 2001). Kegley et al. (2001) showed that Dioryctria abietivorella (Grote) and L. occidentalis produced the highest level of damage in cones and seeds of another five-needle pine species, whitebark pine (P. albicaulis Engelm.). Schwandt et al. (2010) also reported that cone and seed insects can occasionally cause high losses of cones/seeds in five-needle pines. In the northern range of P. strobiformis in the United States, DePinte et al. (2020) observed damage by D. abietivorella, Eupithecia spermaphaga, C. ponderosae, and L. occidentalis. Similar to the range in Mexico, L. occidentalis was the most common damaging agent (DePinte, 2016). This insect caused damage in almost half of the sampled cones and seed in the present study, and it has the capacity to cause substantial damage. In Europe, L. occidentalis is an invasive species and has been found to cause damage in up to 70% of seeds in natural pine stands (Lesieur et al., 2014). Some other insects found to cause little damage in the present study (for example, M. albifrons damaged 1% of seeds and 6% of cones), also have the potential to cause higher levels of damage and affect reproductive output: Blake et al. (1989) reported a loss of 70% in cones of Pinus ponderosa Doug in northern Arizona, United States, caused by M. albifrons.
Five-needle pines in North America, such as P. strobiformis, are of special concern due to their susceptibility to the non-native invasive tree disease, white pine blister rust (caused by the fungal pathogen C. ribicola) (Geils et al., 2010). White pine blister rust has not yet been documented in Mexico, but occurs just north of the Mexican border in New Mexico (USA), on P. strobiformis (Conklin et al., 2009). Maintaining robust and healthy cone and seed production is critical for enhancing natural regeneration and providing seed for genetic resistance breeding efforts and artificial reforestation (Sniezko et al., 2018; Schoettle et al., 2019). Our findings indicate a need for increased monitoring of cone and seed insect populations and damage, particularly as climate change is increasing tree mortality in Mexico (Sáenz-Romero et al., 2020).
Modeling Seed and Cone Traits and Insect Damage, Based on Environmental Variables Using Regression Machine Learning Algorithms
The percentage of incomplete seeds in P. strobiformis at the tree level was correlated with various climate and soil variables and with crown dieback. The most important variables were degree-days >5°C (DD5), GSP, WINP, and SMRPB (Table 3). In a study involving Pinus sylvestris var. Mongolian, He et al. (2018) found that the richness and divergence of the cone traits were positively correlated with water availability, while the seed diversity indices were negatively correlated with water availability. These researchers suggested that pines maximize the characteristics of their seeds in multiple dimensions to make their resources more efficient. Despland and Houle (1997) sampled Pinus banksiana serotinous cones produced between 1969 and 1992, observing that reproductive variables were positively associated with high temperatures, while we found that high temperatures were often associated with the percentage damage to incomplete seeds in this study (Table 3). Cain and Shelton (2000) reported that P. taeda seed production was positively correlated with the average monthly precipitation and negatively correlated with temperature.
We found that cone damage by M. albifrons was positively related to the OM content (%), zinc concentration and mean temperature in the warmest month (Table 4). Altieri and Nicholls (2003) also report that soils with a high content of OM and active biology manage better to prevent insect attack. Jamieson et al. (2012) suggest that heat and drought can cause plants to be less tolerant to insects. Several authors report the relationship between zinc and plant health; e.g., Broadley et al. (2007) and Di Baccio et al. (2005) mention that at certain concentrations zinc is toxic, but is an essential component of thousands of plant proteins. Zinc is the second transition metal after iron (Fe) and the only metal represented in the six enzyme classes (Broadley et al., 2007). Tsonev and Cebola Lidon (2012) showed that excess Zn2+ after a broad response to heavy metals (Cd, Mg, Cu) causes necrosis, wilt and a decrease in biomass; Tripathi et al. (2015) mentioned that Zn is a structural constituent or regulatory cofactor for different enzymes and proteins and that optimum culture growth is generally maintained by absorbing Zn in the divalent form. Zinc performs various important functions in plants: (i) it regulates carbonic anhydrase for binding to carbohydrates in plants; (ii) it promotes metabolism of carbohydrates, proteins, auxins, pollen formation; and (iii) it is an essential element in biological membranes and participates in defense mechanisms against harmful pathogens. Lee et al. (2007) concluded that one of the highest antioxidant defense mechanisms in transgenic tall fescue plants is the overexpression of the CuZnSOD and APX genes. Finally, Mihăiescu et al. (2011) and Wu et al. (2015) reported that in plants Zn generates greater resistance to hot and dry climates as well as resistance to bacterial and fungal diseases.
In the seed damaged by L. occidentalis the most important variables were SMRPB, degree-days >5°C (DD5), winter precipitation (WINP), and manganese concentration in the soil (Table 5). Zhu et al. (2014) reported that the north to south limits of distribution in America of L. occidentalis coincide with the limits of the coniferous host plants, which suggests that climate is the main limiting factor. According to Tripathi et al. (2015), manganese is an essential microelement whose bioavailability is affected by the pH (more than 6.5) of the soil and it participates in stress-tolerant mechanisms of higher plants by serving as a cofactor of various antioxidant enzymes. However, St. Clair and Lynch (2005) found that hyperaccumulation of Mn in tree species that grow in acidic soils negatively affects their health. Subrahmanyam and Rathore (2000) indicated that the Mn toxicity can reduce plant photosynthesis.
There is no direct information available about the impact of climate or nutrients on the development of pests in P. strobiformis, or other Mexican pines. The possible relationships or interactions of the attack by M. albifrons on the cones and by L. occidentalis on the seeds were therefore directly inferred from the data obtained.
Conclusion
The information reported in this study is intended to enhance damage measurement parameters, both in the laboratory and in the field, to enable detection of insect damage in P. strobiformis. We confirmed that much of the damage to seeds caused by insects (80%) cannot be detected by visual observation and that analytical tools, such as X-ray techniques, are therefore required to reveal the full extent of the damage. Future research is needed to investigate spatial and temporal patterns of insect activity, population dynamics, and their relationships through time and space with environmental factors. The study findings contribute to predicting some types of insect damage and the proportion of viable seeds and could therefore be used to develop an integrated management programme for P. strobiformis pests.
Data Availability Statement
The original contributions presented in the study are included in the article/Supplementary Material, further inquiries can be directed to the corresponding author/s.
Author Contributions
AL-S and CW conceived and designed the experiments, conducted sampling, analyzed the data, and prepared figures and tables. AL-S and RÁ-Z identified the insect damages. KW and CW contributed reagents, materials, and analysis tools. CW and AL-S wrote the paper. All authors discussed the results, contributed to the article, and approved the submitted version.
Funding
This material is based on work supported by the National Science Foundation under Grants No. EF-1442597. Cone and seed collections in the US were partially financed by the USDA Forest Service Gene Conservation Program. We are also grateful to CONACYT for the financial support provided to AL-S.
Conflict of Interest
The authors declare that the research was conducted in the absence of any commercial or financial relationships that could be construed as a potential conflict of interest.
Acknowledgments
Cone sampling was supported by UJED (Universidad Juárez del Estado de Durango). We are grateful to Sergio Leonel Simental-Rodríguez, Javier Hernández-Velasco, and Carlos Alonso Reyes-Murillo for assistance in collecting cones and field data. Ivan Leal-Sáenz helped to measure cone and seed damage.
Supplementary Material
The Supplementary Material for this article can be found online at: https://www.frontiersin.org/articles/10.3389/fpls.2021.628795/full#supplementary-material
References
Aguirre-Gutiérrez, J., Serna-Chavez, H. M., Villalobos-Arambula, A. R., Pérez de la Rosa, J. A., and Raes, N. (2015). Similar but not equivalent: ecological niche comparison across closely related Mexican white pines. Divers. Distrib. 21, 245–257. doi: 10.1111/ddi.12268
Altieri, M. A., and Nicholls, C. I. (2003). Soil fertility management and insect pests: harmonizing soil and plant health in agroecosystems. Soil Till. Res. 72, 203–211. doi: 10.1016/S0167-1987(03)00089-8
Álvarez-Zagoya, R. (2019). Fotografías Tomadas de Material Entomológico Depositado en la Colección del CIIDIR Unidad Durango (Durango).
Álvarez-Zagoya, R., and Márquez-Linares, M. A. (1994a). “Factores de mortalidad de conos de Pinus cooperi en San Dimas, Dgo,” in Memorias del XXIX Congreso Nacional de Entomología (México: Sociedad Mexicana de Entomología), 245–246.
Álvarez-Zagoya, R., and Márquez-Linares, M. A. (1994b). Aplicación de Tabla de Vida Para Conos de Pinus cooperi var. ornelasi. Informe Técnico Final, Fase I. Instituto Politécnico Nacional, CIIDIR Unidad Durango, Dgo.
Álvarez-Zagoya, R., and Márquez-Linares, M. A. (1998). “Plagas y enfermedades de las pináceas de Durango,” in Pináceas de Durango, eds A. García-Arévalo and S. González-Elizondo (A.C. Xalapa: Instituto de Ecología), 125–149.
Badnakhe, M. R., Durbha, S. S., Jagarlapudi, A., and Gade, R. M. (2018). Evaluation of Citrus Gummosis disease dynamics and predictions with weather and inversion based leaf optical model. Comput. Electr. Agric. 155, 130–141. doi: 10.1016/j.compag.2018.10.009
Baker, A. S. (1967). Colorimetric determination of nitrate in soil and plant extracts with brucine. J. Agric. Food Chem. 15, 802–806. doi: 10.1021/jf60153a004
Bates, S. L., Lait, C. G., Borden, J. H., and Kermode, A. R. (2001). Effect of feeding by the western conifer seed bug, Leptoglossus occidentalis, on the major storage reserves of developing seeds and on seedling vigor of Douglas-fir. Tree Physiol. 21, 481–487. doi: 10.1093/treephys/21.7.481
Blake, E. A., Wagner, M. R., and Koerber, T. W. (1989). Relative effect of seed and cone insects on ponderosa pine in Northern Arizona. J. Econ. Entomol. 82, 1691–1694. doi: 10.1093/jee/82.6.1691
Bower, A. D., McLane, S. C., Eckert, A., Jorgensen, S., Schoettle, A., and Aitken, S. (2011). “Conservation genetics of high elevation five-needle white pines,” in The future of high-elevation, five-needle white pines in Western North America: Proceedings of the High Five Symposium. 28-30 June 2010; Missoula, MT. Proceedings RMRS-P-63, eds R. E. Keane, D. F. Tomback, M. P. Murray, C. M. Smith (Fort Collins, CO: U.S. Department of Agriculture, Forest Service, Rocky Mountain Research Station), 98–117.
Bracalini, M., Benedettelli, S., Croci, F., Terreni, P., Tiberi, R., and Panzavolta, T. (2013). Cone and seed pests of Pinus pinea: assessment and characterization of damage. J. Econ. Entomol. 106, 229–234. doi: 10.1603/EC12293
Bramlett, D. L., Belcher, E. W Jr., Debarr, G. L, Hertel, G. D., Karrfalt, R. P., et al. (1977). Cone Analysis of Southern Pines- A Guidebook. General Technical Report SE 13. Asheville, NC: U.S. Department of Agriculture, Forest Service, Southeastern Forest Experiment Station.
Broadley, M. R., White, P. J., Hammond, J. P., Zelko, I., and Lux, A. (2007). Zinc in plants. New Phytol. 173, 677–702. doi: 10.1111/j.1469-8137.2007.01996.x
Bustamante-García, V., Prieto-Ruíz, J. A., Álvarez-Zagoya, R., Carrillo-Parra, A., Corral-Rivas, J. J., and Merlín-Bermudes, E. (2012). Factors affecting seed production of Pinus engelmannii Carr in seed stands in Durango State, Mexico. Southwestern Entomol. 37, 351–360. doi: 10.3958/059.037.0311
Cain, M. D., and Shelton, M. G. (2000). Revisiting the relationship between common weather variables and loblolly–shortleaf pine seed crops in natural stands. New For. 19, 187–204. doi: 10.1023/A:1006624907975
Castellanos, J. Z., Uvalle-Bueno, J. X., and Aguilar-Santelises, A. (1999). Memoria del Curso Sobre Interpretación de Análisis de Suelos, Agua Agrícolas, Plantas y ECP. México: Instituto para la Innovación Tecnológica en la Agricultura. p. 188.
Chavagnat, A. (1985). Use of soft X-ray radiography for studying seed quality in horticulture. Seed Res. Horticult. 215, 153–158. doi: 10.17660/ActaHortic.1987.215.20
Choudhuri, D. K., and Banerjee, S. (1975). Qualitative and quantitative composition of acari and collembola in relation to soil organic matter-microbes complex. Orient. Insects 9, 313–316. doi: 10.1080/00305316.1975.10434500
Cibrián-Tovar, D., Ebel, B. H., Yates, H. O. III., and Méndez-Montiel, J. T. (1986). Insectos de Conos y Semillas de las Coníferas de México. Cone and Seed Insects of the Mexican Conifers. U.S. Dept. Agr. Gen. Tech. Rep. SE-40. Departament of Agriculture, Forest Service, Southern Forest Experiment Station. Asheville, NC, USA, p. 110. doi: 10.2737/SE-GTR-40
Cibrián-Tovar, D., Méndez-Montiel, J. T., Campos-Bolaños, R., Yates, H. O. III., and Flores-Lara, J. (1995). Insectos Forestales de México/Forest Insects of Mexico. Universidad Autónoma Chapingo. Texcoco, Edo de Méx., México/USDA Forest Service. Asheville, NC. USA. p. 453.
CONAFOR (2011). Inventario Nacional Forestal y de Suelos: Manual y Procedimientos Para el Muestreo de Campo Re-Muestreo. Comisión Nacional Forestal (Conafor). Available online at: https://www.climateactionreserve.org/wp-content/uploads/2011/03/Sampling_Manual-_Remuestreo-_Conafor_INFyS.pdf
Conklin, D. A., Fairweather, M., Ryerson, D., Geils, B., and Vogler, D. (2009). White Pines, Blister Rust, and Management in the Southwest. USDA Forest Service, Southwestern Region, R3-FH-09-01. p. 16. Available online at: https://www.fs.fed.us/psw/publications/vogler/psw_2009_vog~ler001_conklin.pdf
Cushman, S. A., and Wallin, D. O. (2002). Separating the effects of environmental, spatial and disturbance factors on forest community structure in the Russian Far East. For. Ecol. Manage. 168, 201–215. doi: 10.1016/S0378-1127(01)00744-7
DeBarr, G. L. (1970). Characteristics and radiographic detection of seed bug damage to slash pine seed. Florida Entomol. 53, 109–117. doi: 10.2307/3493454
Del Río-Mora, A. (1980). Identificación de las principales plagas de conos de Pinus spp. del Campo Experimental “Barranca de Cupatitzio”. Uruap, Mich. Cienc. Forest. 5, 17–42.
DePinte, D. E. (2016). Cone and Seed Insects of Southwestern White Pine, Pinus strobiformis, in Arizona, New Mexico, and Texas. Master of Forestry Professional Paper. School of Forestry, Northern Arizona University.
DePinte, D. E., Waring, K. M., and Gaylord, M. L. (2020). Cone and Seed Insects of Southwestern White Pine. Forest Insect and Disease Leaflet 189. U. S. Deparment of Agriculture. Forest Service. p. 8.
Despland, E., and Houle, G. (1997). Climate influences on growth and reproduction of Pinus banksiana (Pinaceae) at the limit of the species distribution in eastern North America. Am. J. Bot. 84, 928–937. doi: 10.2307/2446283
Di Baccio, D., Kopriva, S., Sebastiani, L., and Rennenberg, H. (2005). Does glutathione metabolism have a role in the defence of poplar against zinc excess? New Phytol. 167, 73–80. doi: 10.1111/j.1469-8137.2005.01462.x
Dormont, L., Roques, A., and Trosset, L. (1996). Insect damage to cones and other mortality factors limiting natural regeneration potential of Swiss stone pine (Pinus cembra L.) in the northern French Alps. Ann. Sci. Forest. 53, 153–158. doi: 10.1051/forest:19960112
Dunn, D., and Crutchfield, J. P. (2006). Insects, Trees, and Climate: The Bioacoustic Ecology of Deforestation and Entomogenic Climate Change. Santa Fe Institute Working paper New Mexico, 06:12–30. Available online at: //arxiv.org/abs/q-bio/0612019
Fairweather, M. L., McMillin, J., Rogers, T., Conklin, D., and Fitzgibbon, B. (2006). Field Guide to Insects and Diseases of Arizona and New Mexico Forests. USDA Forest Service Southwestern, Region MR-R3-16-3, p. 215.
Farjon, A., and Styles, B. T. (1997). Pinus (pinaceae). Flora Neotropica. Monograph 75. Bronx, NY: The New York Botanical Garden.
Geils, B. W., Hummer, K. E., and Hunt, R. S. (2010). White pines, Ribes, and blister rust: a review and synthesis. Forest Pathol. 40, 147–85. doi: 10.1111/j.1439-0329.2010.00654.x
Gnanadesikan, R., Kettenring, J. R., and Tsao, S. L. (1995). Weighting and selection of variables for cluster analysis. J. Classific. 12, 113–136. doi: 10.1007/BF01202271
Guo, F., Zhang, L., Jin, S., Tigabu, M., Su, Z., and Wang, W. (2016). Modeling anthropogenic fire occurrence in the boreal forest of China using logistic regression and random forests. Forests 7:250. doi: 10.3390/f7110250
Hanewinkel, M. (2005). Neural networks for assessing the risk of windthrow on the forest division level — a case study in south-west Germany. Eur. J. For. Res. 124, 243–249. doi: 10.1007/s10342-005-0064-8
Hawryło, P., Bednarz, B., Wezyk, P., and Szostak, M. (2018). Estimating defoliation of Scots pine stands using machine learning methods and vegetation indices of Sentinel-2. Eur. J. Remote Sens. 51, 194–204. doi: 10.1080/22797254.2017.1417745
He, P., Fontana, S., Sui, X., Gessler, A., Schaub, M., Rigling, A., et al. (2018). Scale dependent responses of pine reproductive traits to experimental and natural precipitation gradients. Environ. Exp. Bot. 156, 62–73. doi: 10.1016/j.envexpbot.2018.08.028
Hedlin, A. F., Yates, H. O. III., Cibrián-Tovar, D., Ebel, B. H., Koerber, T. W., and Merkel, E. P. (1980). Cone and Insects of North American Conifers. México: Canadian Forestry Service, United States Forest Service, and Secretaría de Agricultura y Recursos Hidráulicos. p. 122.
Herbert, V. F. (1992). Prácticas de Relaciones Agua-Suelo-Planta-Atmósfera. Universidad Autónoma de Chapingo. Texcoco, México. 167p.
Himanen, K., Helenius, P., Ylioja, T., and Nygren, M. (2015). Intracone variation explains most of the variance in Picea abies seed weight: implications for seed sorting. Canad. J. For. Res. 46, 470–477. doi: 10.1139/cjfr-2015-0379
Jamieson, M. A., Trowbridge, A. M., Raffa, K. F., and Lindroth, R. L. (2012). Consequences of climate warming and altered precipitation patterns for plant-insect and multitrophic interactions. Plant Physiol. 160, 1719–1727. doi: 10.1104/pp.112.206524
Jarvis, A., Reuter, H. I., Nelson, A., and Guevara, E. (2008). Hole-Filled SRTM for the GLOBE VERSION 4, Available from the CGIAR-CSI SRTM 90m Database. Available online at: http://srtm.csi.cgiar.org (accessed April 14, 2020).
Kegley, S., Sturdevant, N., Stein, J., Willhite, B., Flanagan, P., Weatherby, J., et al. (2001). Cone and seed insects and their impact on whitebark pine. Forest Health Protection. Report-01–6, Northern Region, USDA Forest Service, Missoula, Montana. Available online at: https://ir.library.oregonstate.edu/concern/defaults/n583xw229
Kelly, D., and Sork, V. L. (2002). Mast seeding in perennial plants: Why, how, where? Annu. Rev. Ecol. Syst. 33, 427–447. doi: 10.1146/annurev.ecolsys.33.020602.095433
Kingsolver, J. G., Arthur Woods, H., Buckley, L. B., Potter, K. A., MacLean, H. J., and Higgins, J. K. (2011). Complex life cycles and the responses of insects to climate change. Integr. Comp. Biol. 51, 719–732. doi: 10.1093/icb/icr015
Klapwijk, M. J., Csóka, G., Hirka, A., and Björkman, C. (2013). Forest insects and climate change: long-term trends in herbivore damage. Ecol. Evol. 3, 4183–4196. doi: 10.1002/ece3.717
Knudsen, D., Peterson, G. A., and Pratt, P. F. (1982). Lithium, sodium, and potassium. Methods Soil Anal. 2 Chem. Microbiol. Prop. 9, 225–246. doi: 10.2134/agronmonogr9.2.2ed.c13
Krogh, A. (2008). What are artificial neural networks? Nat. Biotechnol. 26, 195–197. doi: 10.1038/nbt1386
Kruskal, W. H., and Wallis, W. A. (1952). Use of ranks in one-criterion variance analysis. J. Am. Stat. Assoc. 47, 583–621. doi: 10.1080/01621459.1952.10483441
Krzanowski, W. J. (1987). Selection of variables to preserve multivariate data structure, using principal components. Appl. Stat. 36, 22–33. doi: 10.2307/2347842
Kuhn, M. (2012). Variable Importance Using the Caret Package. Available online at: https://cran.cermin.lipi.go.id/web/packages/caret/vignettes/caretSelection.pdf (accessed May 04, 2020).
Lanyon, L. E., and Heald, W. R. (1982). Magnesium, calcium, strontium, and barium. Methods Soil Anal. 2 Chem. Microbiol. Prop. 9, 247–262. doi: 10.2134/agronmonogr9.2.2ed.c14
Leal-Sáenz, A., Waring, K. M., Menon, M., Cushman, S. A., Eckert, A., Flores-Renteria, L. L., et al. (2020). Morphological differences in Pinus strobiformis across latitudinal and elevational gradients. Front. Plant Sci. 11:559697. doi: 10.3389/fpls.2020.559697
Lee, S. H., Ahsan, N., Lee, K. W., Kim, D. H., Lee, D. G., Kwak, S. S., et al. (2007). Simultaneous overexpression of both CuZn superoxide dismutase and ascorbate peroxidase in transgenic tall fescue plants confers increased tolerance to a wide range of abiotic stresses. J. Plant Physiol. 164, 1626–1638. doi: 10.1016/j.jplph.2007.01.003
León, A. R., and Aguilar, A. S. (1987). “Materia orgánica,” in Análisis Químico Para Evaluar la Fertilidad del Suelo, eds A. Aguilar, J. D. Etchevers, J. Z. Castellanos, (Chapingo: Estado de México, Publicación especial No. 1 Sociedad Mexicana de la Ciencia del Suelo). p. 217.
Lesieur, V., Yart, A., Guilbon, S., Lorme, P., Auger-Rozenberg, M. A., and Roques, A. (2014). The invasive Leptoglossus seed bug, a threat for commercial seed crops, but for conifer diversity? Biol. Invas. 16, 1833–1849. doi: 10.1007/s10530-013-0630-9
Linhart, Y. B., Moreira, X., Snyder, M. A., and Mooney, K. A. (2014). Variability in seed cone production and functional response of seed predators to seed cone availability: support for the predator satiation hypothesis. J. Ecol. 102, 576–583. doi: 10.1111/1365-2745.12231
Lippitt, L., Fidelibus, M. W., and Bainbridge, D. A. (1994). Native seed collection, processing, and storage for revegetation projects in the western United States. Restor. Ecol. 2, 120–131. doi: 10.1111/j.1526-100X.1994.tb00049.x
Looney, C. E., and Waring, K. M. (2013). Pinus strobiformis (southwestern white pine) stand dynamics, regeneration, and disturbance ecology: a review. For. Ecol. Manage. 287, 90–102. doi: 10.1016/j.foreco.2012.09.020
Luther, J. E., Franklin, S. E., Hudak, J., and Meades, J. (1997). Forecasting the susceptibility and vulnerability of Balsam fir stands to insect defoliation with Landsat Thematic Mapper data. Remote Sens. Environ. 59, 77–91. doi: 10.1016/S0034-4257(96)00108-3
Machado, C. F., and Cícero, S. M. (2003). 'Aroeira-branca'[Lithraea molleoides (Vell.) Engl.-Anacardiaceae] seed quality evaluation by the X-ray test. Sci. Agric. 60, 393–397. doi: 10.1590/S0103-90162003000200026
Maronna, R. A., Martin, R. D., Yohai, V. J., and Salibián-Barrera, M. (2018). Robust Statistics: Theory and Methods (With R). New York, NY: Wiley. doi: 10.1002/9781119214656
McColloch, J. W., and Hayes, W. P. (1922). The reciprocal relation of soil and insects. Ecology 3, 288–301. doi: 10.2307/1929431
McGarigal, K., Cushman, S., and Stafford, S. (2000). Multivariate Statistics for Wildlife and Ecology Research. New York, NY: Springer Science. doi: 10.1007/978-1-4612-1288-1
Mendoza, R., and Espinoza, A. (2017). Guía Técnica para Muestreo de Suelos. Obtenido de Universidad Nacional Agraria y Catholic Relief Services. Available online at: http://repositorio.una.edu.ni/3613/1/P33M539.pdf
Mihăiescu, T., Goji, G., and Dinulică, F. (2011). Bioaccumulation of cadmium and zinc in the fruits of some forestry species of sanogenic and economical importance from the area of Copşa Mică. Bull. Transil. Univ. Braşov. II Forest. Wood Eng. Agric. Food. Eng. 4, 59–64. Available online at: https://search.proquest.com/openview/8a59ffa5321529bf3efe09737d0209c1/1?pq-origsite=gscholar&cbl=105973
Mualem, Y. (1976). A new model for predicting the hydraulic conductivity of unsaturated porous media. Water Resour. Res. 12, 513–522. doi: 10.1029/WR012i003p00513
Musolin, D. L. (2007). Insects in a warmer world: ecological, physiological and life-history responses of true bugs (Heteroptera) to climate change. Glob. Chang. Biol. 13, 1565–1585. doi: 10.1111/j.1365-2486.2007.01395.x
Negrón, J. F., McMillin, J. D., Anhold, J. A., and Coulson, D. (2009). Bark beetle-caused mortality in a drought-affected ponderosa pine landscape in Arizona, USA. For. Ecol. Manage. 257, 1353–1362. doi: 10.1016/j.foreco.2008.12.002
Ogris, N., and Jurc, M. (2010). Sanitary felling of Norway spruce due to spruce bark beetles in Slovenia: a model and projections for various climate change scenarios. Ecol. Model. 221, 290–302. doi: 10.1016/j.ecolmodel.2009.05.015
Olsen, S. R., Cole, C. V., Watanabe, F. S, and Dean, L. A. (1954). Estimation of Available Phosphorus in Soil by Extraction with NaHCO3, USDA Ciraza (Quoted from, Method of Soil Analysis, CA black 2nd Edn., Vol. 1165. Madison, WI: Am. Soc. Agron. Inc.
Park, Y., and Chung, Y. (2006). Hazard rating of pine trees from a forest insect pest using artificial neural networks. For. Ecol. Manage. 222, 222–233. doi: 10.1016/j.foreco.2005.10.009
Peacock, L., Worner, S., and Sedcole, R. (2006). Climate variables and their role in site discrimination of invasive insect species distributions. Environ. Entomol. 35, 958–963. doi: 10.1603/0046-225X-35.4.958
Poncet, B. N., Garat, P., Manel, S., Roques, A., and Despres, L. (2009). The effect of climate on masting in the European larch and on its specific seed predators. Oecologia 159, 527–537. doi: 10.1007/s00442-008-1233-5
Pureswaren, D. S., Roques, A., and Battisti, A. (2018). Forest insects and climate change. Curr. For. Rep. 4, 35–50. doi: 10.1007/s.40725-018-0075-6
R Development Core Team (2017). R: A Language and Environment for Statistical Computing. Vienna: R Foundation for Statistical Computing. Available online at: https://www.R-project.org/ (accessed October 03, 2018).
Richardson, D. M. (2000). Ecology and Biogeography of Pinus. Cambridge, UK: Cambridge University Press.
Ruth, D. S., Miller, G. E., and Sutherland, J. R., (eds.). (1982). A Guide to the Common Insect Pests and Diseases in Spruce Seed Orchards in British Columbia. Information Report Canadian Forestry Service (Canada). Victoria, BC: Canadian Forest Service, Pacific Forest Research Centre, 26.
Sáenz-Romero, C., Mendoza-Maya, E., Gómez-Pineda, E., Blanco-García, A., Endara-Agramont, A. R., Lindig-Cisneros, R., et al. (2020). Recent evidence of Mexican temperate forest decline and the need for ex situ conservation, assisted migration, and translocation of species ensembles as adaptive management to face projected climatic change impacts in a megadiverse country. Canad. J. For. Res. 50, 843–854. doi: 10.1139/cjfr-2019-0329
Salas, E. A. L., Valdez, R., and Michel, S. (2017). Summer and winter habitat suitability of Marco Polo argali in southeastern Tajikistan: a modeling approach. Heliyon 3:e00445. doi: 10.1016/j.heliyon.2017.e00445
Salinas-Moreno, Y., Ager, A., Vargas, C. F., Hayes, J. L., and Zúniga, G. (2010). Determining the vulnerabilty of Mexican pine forests to bark beetles of the genus Dendroctonus Ehrichson (Coleoptera: Curculionidae: Scolytinae). For. Ecol. Manage. 260, 52–61. doi: 10.1016/j.foreco.2010.03.029
Samano, S., and Tomback, D. F. (2003). Cone opening phenology, seed dispersal, and seed predation in southwestern white pine (Pinus strobiformis) in southern Colorado. Écoscience 10, 319–326. doi: 10.1080/11956860.2003.11682780
Schmid, J. M., Mitchell, J. C., Carlin, K. D., and Wagner, M. R. (1984). Insect damage, cone dimensions, and seed production in crown levels of ponderosa pine. Great Basin Natur. 44, 575–758.
Schoettle, A. W., Jacobi, W. R., Waring, K. M., and Burns, K. S. (2019). Regeneration for resilience framework to support regeneration decisions for species with populations at risk of extirpation by white pine blister rust. New Forests 50, 89–114. doi: 10.1007/s11056-018-9679-8
Schwandt, J. W., Lockman, I. B., Kliejunas, J. T., and Muir, J. A. (2010). Current health issues and management strategies for white pines in the western United States and Canada. Forest Pathol. 40, 226–250. doi: 10.1111/j.1439-0329.2010.00656.x
Seidl, R., Fernandes, P. M., Fonseca, T. F., Gillet, F., Jönsson, A. M., Merganičová, K., et al. (2011). Modelling natural disturbances in forest ecosystems: a review. Ecol. Modell. 222, 903–924. doi: 10.1016/j.ecolmodel.2010.09.040
Shirk, A. J., Cushman, S. A., Waring, K. M., Wehenkel, C., Leal-Sáenz, A., Toney, C., et al. (2018). Southwestern white pine (Pinus strobiformis) species distribution models project a large range shift and contraction due to regional climatic changes. For. Ecol. Manage. 411, 176–186. doi: 10.1016/j.foreco.2018.01.025
Sniezko, R. A., Kegley, A., Jacobs, J., Danchok, R., Luis, B., Long, S., et al. (2018). “Variation in blister rust resistance and early height growth in three populations of southwestern white pine (Pinus strobiformis) and implications for management and conservation,” in Proceedings of the IUFRO Joint Conference: Genetics of Five-Needle Pines, Rusts of Forest Trees, and Strobusphere; 2014 June 15–20; Fort Collins, CO. Proc. RMRS-P-76, A. W. Schoettle, R. A. Sniezko, J. T. Kliejunas (Fort Collins, CO: US Department of Agriculture, Forest Service, Rocky Mountain Research Station), 140–148.
St. Clair, S. B., and Lynch, J. P. (2005). Element accumulation patterns of deciduous and evergreen tree seedlings on acid soils: implications for sensitivity to manganese toxicity. Tree Physiol. 25, 85–92. doi: 10.1093/treephys/25.1.85
Subrahmanyam, D., and Rathore, V. S. (2000). Influence of manganese toxicity on photosynthesis in ricebean (Vigna umbellata) seedlings. Photosynthetica 38, 449–453. doi: 10.1023/A:1010998226323
Tripathi, D. K., Singh, S., Singh, S., Mishra, S., Chauhan, D. K., and Dubey, N. K. (2015). Micronutrients and their diverse role in agricultural crops: advances and future prospective. Acta Physiol. Plant. 37, 1–14. doi: 10.1007/s11738-015-1870-3
Tsonev, T., and Cebola Lidon, F. J. (2012). Zinc in plants-an overview. Emir. J. Food Agric. 24, 322–333. Available online at: https://www.researchgate.net/profile/T-Tsonev/publication/267030914_Zinc_in_plants_-_An_overview/links/54ad28a20cf24aca1c6ceabb/Zinc-in-plants-An-overview.pdf
Turgeon, J. J., Roques, A., and de Groot, P. (1994). Insect fauna of coniferous seed cones: diversity, host plant interactions, and management. Annu. Rev. Entomol. 39, 179–212. doi: 10.1146/annurev.en.39.010194.001143
Vázquez, A. A., and Bautista, N. (1993). Guía Para Interpretar el Análisis Químico de Suelo y Agua. México: Departamento de Suelos. Universidad. Autónoma de Chapingo (No. Folleto 1049) p. 29.
Venables, W. N., and Ripley, B. D. (1999). “Chapter 10: Tree-based methods,” in Modern Applied Statistics with S-PLUS, 3rd Edn., Statistics and Computing., eds J. Chambers, W. Eddy, W. Härdle, S. Sheather, and L. Tierney (New York, NY: Springer-Verlag Press), 303–327. doi: 10.1007/978-1-4757-3121-7_10
Villagómez-Loza, M. A., Bello-González, M. A., and Isarain-Chávez, E. (2014). Pinus strobiformis Engelmann: nueva localidad para guanajuato, México. Agrociencia 48, 615–625. Available online at: http://www.scielo.org.mx/pdf/agro/v48n6/v48n6a4.pdf
Villalobos-Arámbula, A. R., Pérez de la Rosa, J. P., Arias, A., and Rajora, O. P. (2014). Cross-species transferability of eastern white pine (Pinus strobus) nuclear microsatellite markers to five Mexican white pines. Genet. Mol. Res. 13, 7571–7576. doi: 10.4238/2014.September.12.24
Vittinghoff, E., and McCulloch, C. E. (2007). Relaxing the rule of ten events per variable in logistic and Cox regression. Am. J. Epidemiol. 165, 710–718. doi: 10.1093/aje/kwk052
Whitehouse, C. M., Roe, A. D., Strong, W. B., Evenden, M. L., and Sperling, F. A. H. (2011). Biology and management of North American cone-feeding Dioryctria species. Can. Entomol. 143, 1–34. doi: 10.4039/n10-045
Wickman, B. E. (1992). Forest Health in the Blue Mountains: The Influence of Insects and Diseases, Vol. 295, ed T. M. Quigley (USDA Forest Service, Pacific Northwest Research Station). doi: 10.2737/PNW-GTR-295
Williams, C. K., Engelhardt, A., Cooper, T., Mayer, Z., Ziem, A., Scrucca, L., et al. (2018). Package ‘caret’, version 6.0-80. Available online at: https://cran.r-project.org/web/packages/caret/caret.pdf (accessed May 14, 2020).
Wu, S., Hu, C., Tan, Q., Li, L., Shi, K., Zheng, Y., et al. (2015). Drought stress tolerance mediated by zinc-induced antioxidative defense and osmotic adjustment in cotton (Gossypium hirsutum). Acta Physiol. Plant. 37:167. doi: 10.1007/s11738-015-1919-3
Wulder, M. A., White, J. C., Bentz, B., Alvarez, M. F., and Coops, N. C. (2006). Estimating the probability of mountain pine beetle red-attack damage. Remote Sens. Environ. 101, 150–166. doi: 10.1016/j.rse.2005.12.010
Keywords: X-ray, stereomicroscopic analysis, seed production, machine learning, regression analyses
Citation: Leal-Sáenz A, Waring KM, Álvarez-Zagoya R, Hernández-Díaz JC, López-Sánchez CA, Martínez-Guerrero JH and Wehenkel C (2021) Assessment and Models of Insect Damage to Cones and Seeds of Pinus strobiformis in the Sierra Madre Occidental, Mexico. Front. Plant Sci. 12:628795. doi: 10.3389/fpls.2021.628795
Received: 16 November 2020; Accepted: 30 March 2021;
Published: 29 April 2021.
Edited by:
Henry D. Adams, Washington State University, United StatesReviewed by:
Daniel Moya, University of Castilla-La Mancha, SpainDirk Vanderklein, Montclair State University, United States
Copyright © 2021 Leal-Sáenz, Waring, Álvarez-Zagoya, Hernández-Díaz, López-Sánchez, Martínez-Guerrero and Wehenkel. This is an open-access article distributed under the terms of the Creative Commons Attribution License (CC BY). The use, distribution or reproduction in other forums is permitted, provided the original author(s) and the copyright owner(s) are credited and that the original publication in this journal is cited, in accordance with accepted academic practice. No use, distribution or reproduction is permitted which does not comply with these terms.
*Correspondence: Christian Wehenkel, wehenkel@ujed.mx